As we discussed in our previous blog, “Transitioning To Precision Medicine,” precision medicine is poised to transform medicine as we’ve known it, from the earliest R&D efforts by drug developers all the way to patient assessment and physician prescribing. In fact, it is projected that the precision medicine market will reach into the hundreds of billions of dollars in the coming years.(1) But getting there presents some of the most challenging data science and interdisciplinary collaboration demands we’ve ever seen.
Unprecedented Data Science and Collaboration Demands
While patient and prescriber may first come to mind when thinking about precision medicine, the diversity of people working toward this progressive approach to medicine is really quite stunning. For example:
Life science researchers are uncovering disease mechanisms and identifying diagnostic and prognostic biomarkers that can help assess individual patient needs, as well as guide clinical drug development.
Genomics companies are making sequencing data more accessible by lowering costs and increasing throughput, while pharmacogenomics experts are deciphering how those data relate to the way patients respond to drugs, and other “omics” researchers are exploring the impact of additional biological processes beyond the genome.
Imaging and AI tools are aiding in the analysis of patient’s medical scans with accuracy and speed not possible through manual assessment.
Diagnostics and medtech companies are creating tests, equipment, and wearable devices to provide real-time metrics on patients and their environments.
Informatics and electronics-health-records (ELH) companies are creating solutions to collect, combine, and securely manage multimodal patient data.
Regulators, policymakers, and insurers are navigating red-tape and incentivizing change.
Truly Integrated Biomedical Data
With so many contributing elements, large volumes of diverse data comprise patients’ ever-evolving health profiles. The figure below was created by researchers at Yale School of Medicine, Harvard Medical School, and Scripps Research Translational Institute to illustrate the complex interplay between the diverse biomedical data modalities available today and the many opportunities for putting the data to use.(2)
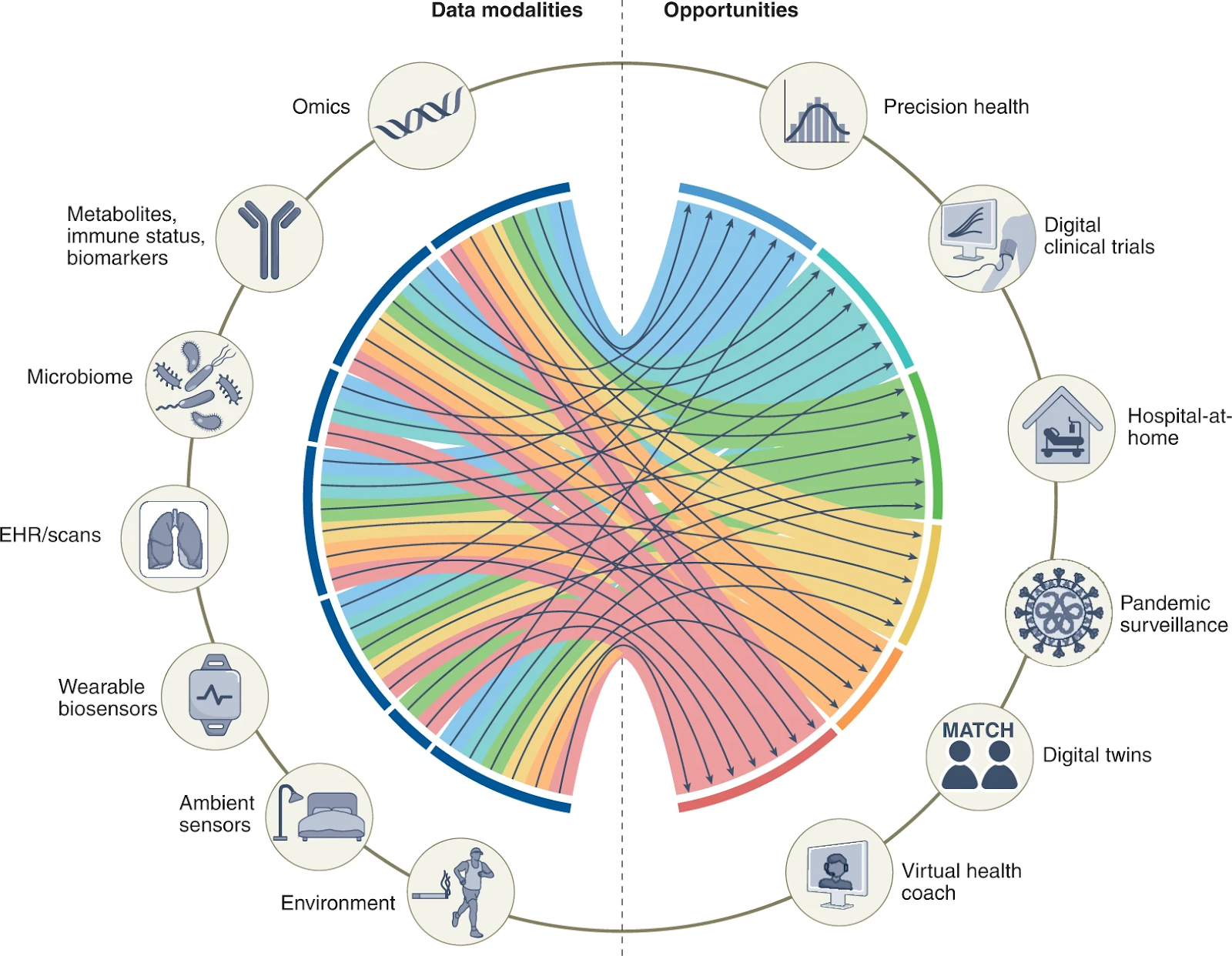
Figure 1: Biomedical data modalities and opportunities for their application.
(Acosta, J.N., Falcone, G.J., Rajpurkar, P. and Topoal, E.J. Nature Medicine [28] 2022.)
All these data points fit together like pieces of a puzzle. Except these data aren’t stagnant, and biological context dictates everything, so the puzzle pieces can shift. Therefore, attaining a clear and actionable picture is an iterative and recursive process. This is a daunting ask, even for companies with strong data infrastructures and data science expertise. Unfortunately, many healthcare organizations feel ill-prepared to take on precision medicine programs for a variety of reasons, from data-science preparedness to IT readiness to skills gaps in analytics, programming and specialized science.(3) In fact, many companies are already struggling to achieve efficiency under the current paradigm and a shift toward personalized medicine will only increase the need to adopt advanced data technologies and fully leverage the data at hand.
Multi-Omics Data
To demonstrate the scope of the data challenges presented by precision medicine, let’s dive deeper into just one of the many data modalities noted in Figure 1—omics data. As illustrated in Figure 2, multi-omics data are the collective data from different “omics'' research areas, such as genomics, epigenomics, transcriptomics, proteomics, metabolomics and phenomics. These data originate from many different assay types and experiments and span many different scales of time and space. And while genomics has long been a primary focus in precision medicine, other areas of research are increasingly being used to help attain a more complete picture of how an individual’s complex biology impacts their health profile.
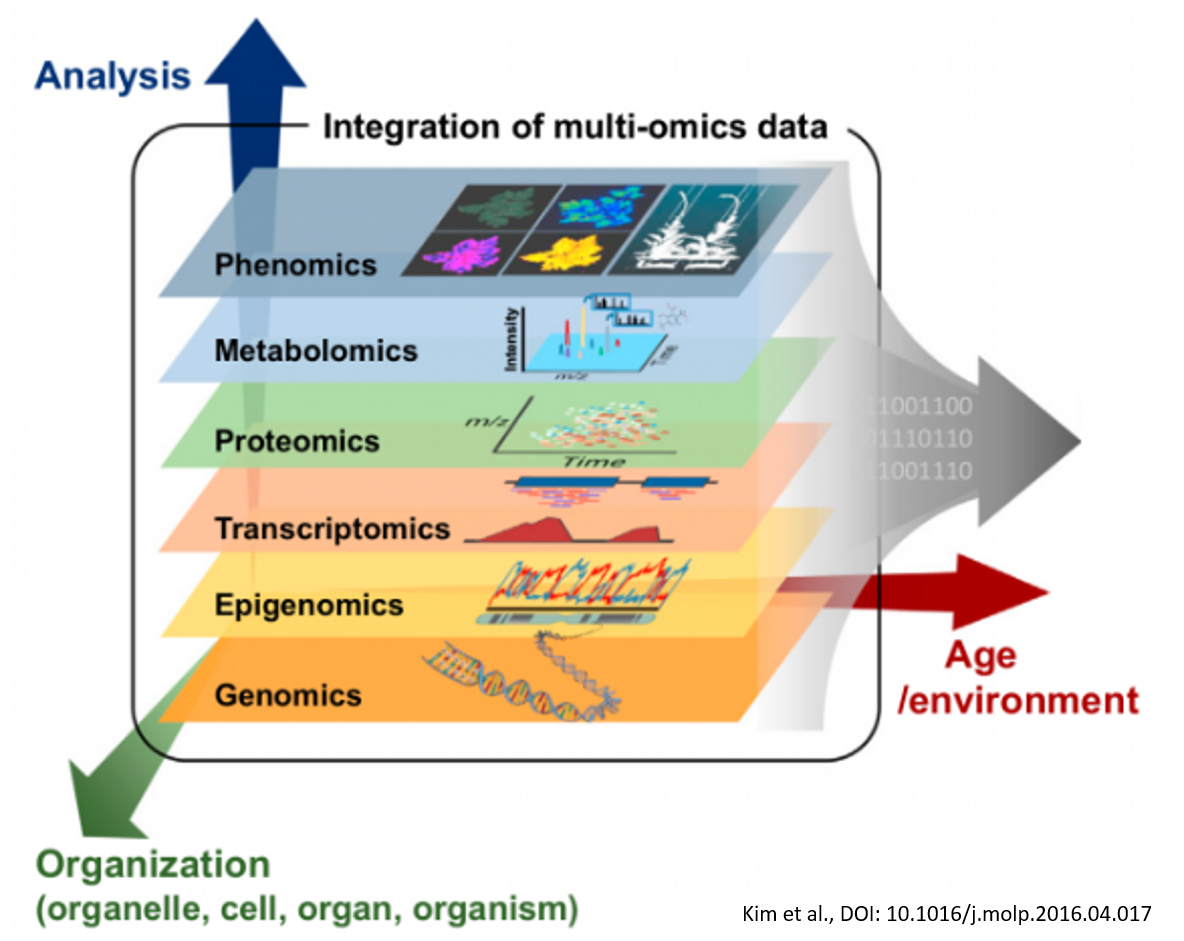
Figure 2: Multi-omics data can help provide a more complete picture of how an individual’s complex biology impacts their health profile. (Kim et. al, Mol. Plant. [9] 2016)
To understand why multi-omics data are so important, think of it this way: Even under the current paradigm, when you’re sick, your doctors don’t just look at a single health parameter, they look at many.(5) What are your symptoms? Have you been exposed to any pathogens? Are you in a high-risk category? How are your vitals? What lab test should be ordered? Etcetera, etcetera. Multi-omics research is about doing the same thing at the cellular level to gain a deeper understanding of disease mechanisms and drug response. So instead of just looking at the static genome alone, we’re looking at multiple dynamic factors across various cells and events in order to scrutinize potentially relevant cellular processes, such as regulatory networks or cell signaling pathways of interest.(6)
As the National Human Genome Research Institute (NHGRI) explains, “While single ‘omic analyses have produced valuable insights, recent studies have shown that integrative (or multi-omic) analysis approaches can improve the classification of disease into clinically relevant subgroups and potentially identify biomarkers of health or disease. Multi-omic analyses can also help define relationships among omic data types to unravel biological networks regulating transitions from health to disease.(7) The NHGRI is leading a multi-omics initiative that will “produce consensus approaches, best practices, and standards that can be generalized across diseases and populations. It will also generate a standardized and harmonized dataset for general research use available through controlled-access processes as well as a portal for visualization. Ultimately, this program will enhance the utility of ‘omic technologies in understanding the biology of health and disease.”(7)
Certainly, a major hurdle to multi-omic research is the multitude of research groups, data types and technologies involved in the various specialty areas. As one review in Frontiers in Genetics points out, “The mushrooming of a myriad of tools, datasets, and approaches tends to inundate the literature and overwhelm researchers new to the field."(8)
Proteomics: One multi-omic example
By digging a bit farther into just one of these “omics”—let’s say proteomics—we can show how the data problem just keeps snowballing. Figure 3 was created by a group of proteomics researchers to provide a high-level summary of the assay and mass spec technologies, quantitative analysis workflows, and data integration requirements that go into making proteomics data actionable in a clinical setting. Each of the individual elements is incredibly complicated on its own. As noted by the authors, marrying all these things together will demand substantial work, but that work is worthwhile because proteomics holds incredible promise to complement genetics and “provide insight about the dynamic behavior of proteins as they represent intermediate phenotypes.”(9)
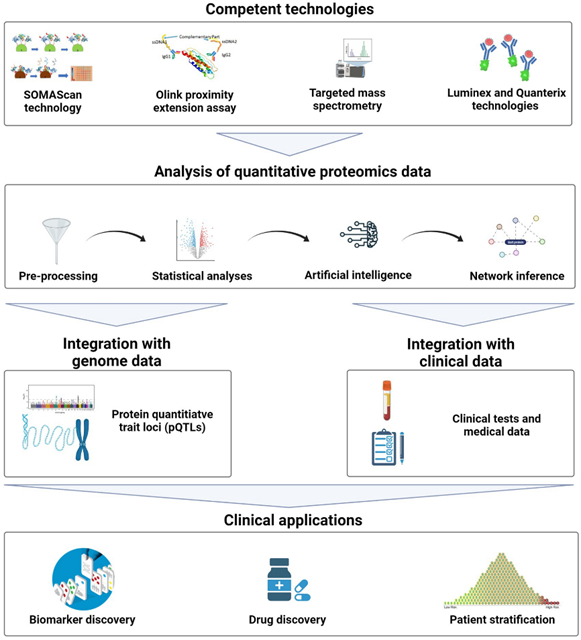
Figure 3: General workflow for applying quantitative proteomics in the clinical setting.
(Rojo, A.C., Heylen, D., Aerts, J. et al. Front Phyisol. [12] 2021)
While proteomics represents just one example, it is safe to say that multi-omics research overall creates incredible demand for an R&D data platform that prioritizes data flow and not just workflow. Research teams will need an infrastructure that can integrate the various specialty tools needed for different types of -omics research, while also helping to harmonize the varied data coming from those tools and ensure those data are FAIR and ready for deeper analysis using predictive modeling tools that will uncover key connections between cellular processes and patient experiences.
Data Technology is Key to Successful Precision Medicine
Our quick review of multi-omics research, and specifically proteomics, has only scratched the surface on the complexity of the technology, data, and collaboration demands presented by precision medicine. Imagine how these demands will blossom when adding in other areas of “omics” research, and then on top of that adding all the additional types of multimodal biomedical and patient data that need to be considered.
One thing is clear—data technology will be key to making precision medicine a reality. According to a recent Forbes article contributed by Weill Cornell Medicine, “There are around 10,000 diseases that affect humans – each of whom, it should be noted, currently generate around 80 megabytes each year in imaging and electronic medical record data. Powerful technology is critical for precision medicine, because the numbers are not on the side of personalization.”(10)
Even in cancer, where precision medicine first gained its footing and has delivered some life-saving breakthroughs for individual patients, the overall impact is mixed.(11-14) A 2018 report from JAMA Oncology indicates that only a small fraction of cancer patients is eligible for precision medications, and of all eligible patients, the overall health impact is often modest.(11-12) A 2021 single-institution retrospective analysis of patients with tumors who underwent genetic variation testing showed more positive results with a disease control rate of around 41%.(13) In an environment where cost is also an influencing factor for patients, policy makers, and insurers, continued improvement of both patient-treatment odds and overall cost-benefit-payoff is imperative. A key way of getting there will be positioning ourselves to best use all the data we have at our disposal.
While, for now, the cumulative effect of precision medicine may be more of a trickle than a flood, work to improve its impact, not just in cancer but in a wide range of conditions, is multifaceted. Aside from a trend toward multi-omics research and more comprehensive data-rich patient profiles, there is work being done to translate all that data into practice. For example, statisticians at the University of North Carolina at Chapel Hill and North Carolina State University at Raleigh have built statistical models to help formalize an optimal dynamic treatment regime.(15) The models comprise a sequence of decision rules, one per decision point, which map patient information to a recommended action, such prescribing a certain drug at a specific dose or time. The complexity of these statistical models demonstrates the complexity of the data at play.
While we may have a long road ahead until precision medicine becomes standard care, companies like Dotmatics are working to create solutions that help researchers harmonize their data and science and move the dial forward.
See how you can leverage the ability to contextualize your findings with data intelligence software, so you can translate your data into practice.
References
Global market for personalized medicine from 2015 to 2022, by product (in billion U.S. dollars). Statista estimates; Grand View Research; ID 728117
Acosta, J.N., Falcone, G.J., Rajpurkar, P. et al. Multimodal biomedical AI. Nat. Med. (28) 2022. https://doi.org/10.1038/s41591-022-01981-2
Percentage of healthcare organizations which are equipped for personal medicine programs as of 2016. Oxford Economics; SAP; ID 726859.
Kim, J., Woo, H.R., and Nam, H.G. Toward Systems Understanding of Leaf Senescence: An Integrated Multi-Omics Perspective on Leaf Senescence Research. Molecular Plant. (9) 2016. https://doi.org/10.1016/j.molp.2016.04.017
Zhang, Y. Why Multi-Omics Is The Future Of Biological Analysis. Forbes. October 22, 2021. [Accessed April 13, 2023]
Carkett, M. and Mishra, T. Beyond the Human Genome: What Is Multi-omics? Tony Blair Institute for Global Change. October 4, 2021. [Accessed April 13, 2023]
Multi-Omics for Health and Disease (Multi-Omics). National Human Genome Research Institute. [Accessed April 13, 2023]
Krassowski, M., Das, V., Sahu, S.K., and Misra, B.B. State of the Field in Multi-Omics Research: From Computational Needs to Data Mining and Sharing. Front. Genet. (11) 2020. https://doi.org/10.3389/fgene.2020.610798
Rojo, A.C., Heylen, D. Aerts, J. et al. Towards Building a Quantitative Proteomics Toolbox in Precision Medicine: A Mini-Review. Front. Genet. (12) 2021. https://doi.org/10.3389/fphys.2021.723510
The Next Life-Saving Innovation in Medicine May Be Developed Only for You: Meet the physician-scientists at Weill Cornell Medicine combating disease with extreme precision. Forbes. [Accessed April 13, 2023].
Cutler, D. Early Returns from the Era of Precision Medicine. JAMA Forum – Drug Pricing. November 20, 2019.
Marquart, B.A., Chen, E.Y., and Prasad, V. Estimation of the Percentage of US Patients With Cancer Who Benefit From Genome-Driven Oncology. JAMA Oncology. 4(8) 2018. doi:10.1001/jamaoncol.2018.1660
Quinn, R., Ratel, R., Sison, C. et al. Impact of Precision Medicine on Clinical Outcomes: A Single-Institution Retrospective Study. Front. Oncol. (11) 2021. https://doi.org/10.3389/fonc.2021.659113
Precision or Personalized Medicine. American Cancer Society. March 30, 2023. [Accessed April 13, 2023].
Kosorok, M.R. and Laber, E.B. Precision Medicine. Ann. Rev. Stat. Appl. (6) 2019. https://doi.org/10.1146/annurev-statistics-030718-105251