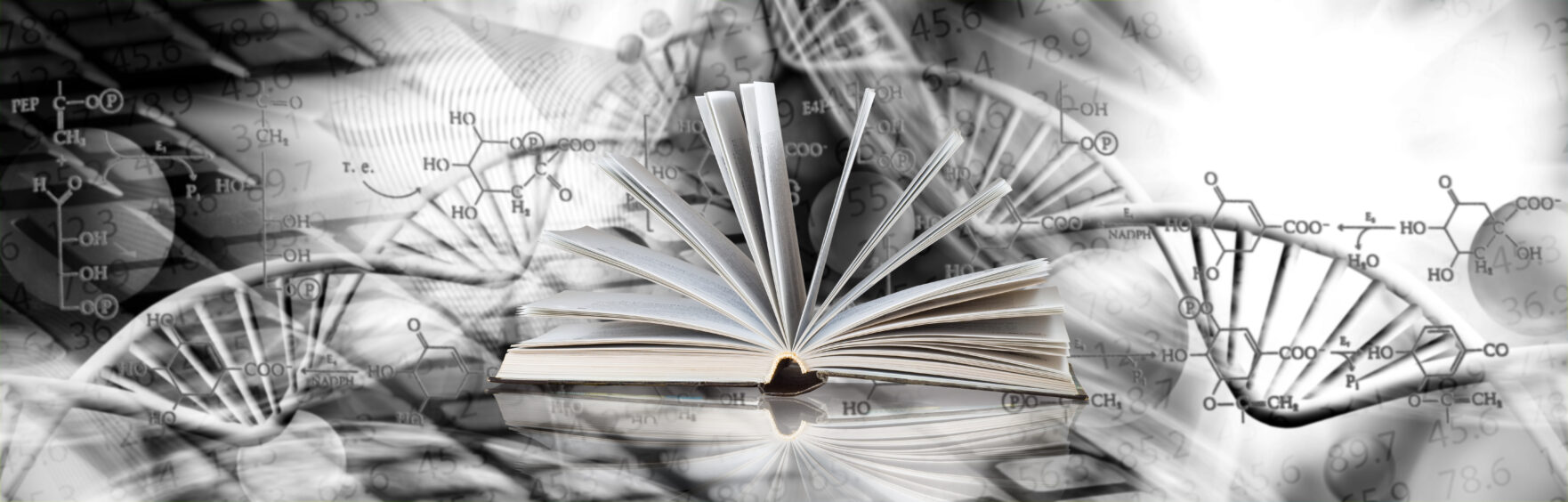
Artificial Neural Networks.
Editor Hugh Cartwright
Methods in Molecular Biology | Springer Protocols
Includes cutting-edge methods and protocols
Provides step-by-step detail essential for reproducible results
Contains key notes and implementation advice from the experts
“This volume presents examples of how Artificial Neural Networks (ANNs) are applied in biological sciences and related areas. Chapters cover a wide variety of topics, including the analysis of intracellular sorting information, prediction of the behavior of bacterial communities, biometric authentication, studies of Tuberculosis, gene signatures in breast cancer classification, the use of mass spectrometry in metabolite identification, visual navigation, and computer diagnosis. Written in the highly successful Methods in Molecular Biology series format, chapters include introductions to their respective topics, application details for both the expert and non-expert reader, and tips on troubleshooting and avoiding known pitfalls.
Authoritative and practical, Artificial Neural Networks: Third Edition should be of value to all scientists interested in the hands-on application of ANNs in the biosciences.” Available here.
Of course, my recommendation may be slightly biased by the fact that Charles Fracchia, VP of Data at Dotmatics and CEO at BioBright, has contributed a chapter!
Charles’ chapter, Secure and Scalable Collection of Biomedical Data for Machine Learning Applications, covers data collection and labeling as prerequisites to training of any algorithm: data collection with scalability and security being key to avoiding costly, delaying bottlenecks in high-throughput workflows such as high content imaging and sequencing. Maintenance of biomedical data security is hugely important and Charles addresses best practices to achieve security, with a focus on practicality and scalability. He also covers the challenge of obtaining usable, rich metadata from the collected data, often in fragmented and proprietary formats, and how underutilized metadata plays a key role in creating labeled data for use in the training of neural networks.
I know that many of our current customers appreciate being able to discuss digital biosecurity with Charles so it’s great to see his expertise recognized in the wider community.
Enjoy!