Artificial intelligence (AI) is transforming drug discovery and development.
We’ve been hearing this refrain for years. Countless pundits have estimated how and when AI will provide the biopharma industry with much needed process efficiencies and innovation infusion. Lower costs. Faster times to market. Fewer failures. Wider target space. The details of these predictions vary; but the core message remains the same: in silico work can supercharge benchwork in a new-and-improved hybrid model to drive pharma innovation.
With the sweeping hope of an AI-powered future for pharma R&D, it can be easy to gloss over some inevitable truths: that cells, disease pathways, and treatment modalities are incredibly difficult to model, and making predictions necessitates organizing and processing huge volumes of multimodal data; that while AI-first approaches are already working well in some areas of R&D where training data is plentiful, like target identification and drug repurposing, there is much room to grow in other areas, like predicting pharmacokinetics and pharmacodynamics, where refining models with complex cellular-dynamics data is very challenging; that in silico predictions must hold up both in vitro and in vivo, and uniting these worlds is a huge undertaking; and that, ultimately, as the role of benchwork evolves within an AI-driven R&D paradigm so must our lab workflows and technologies.
Digitally Transforming for AI Readiness
The new era of AI-driven R&D will necessitate bridging the in silico world (a dry lab) with the wet lab in order to achieve a Lab-in-a-Loop. A Lab-in-a-Loop requires that all R&D and clinical data be ingested, centralized, and used to train and refine AI models; these models will deliver predictions and insights to inform the next set of necessary lab experiments. This entire process must be iterative and capable of near exponential scale so that models can produce predictions for specific projects and be refined for new projects.
This prospect may seem daunting to companies that, for decades, have pursued single modes of bench-based discovery, thereby leaving their teams, technology, and data siloed. Transitioning to an AI-empowered multimodal approach to R&D will necessitate the collection and preparation of data from different domains of science, where widely different specialty software, tools, and instruments churn out scores of disparate data. Teams will need flexible informatics technology that improves interoperability, eliminates research and data silos, and ensures data is standardized, harmonized, and ready for use within multimodal AI models.
Incremental Digital Coalescence
Creating a Lab-in-a-Loop is not a big-bang moment, but rather an incremental process. Many companies begin their journey by investing in data management technologies that will help them get a better handle on the R&D and clinical data needed to train and refine AI models. Companies with a long history of discipline-based, bench-driven R&D can expect to gradually progress through a digital coalescence whereby they achieve:
Foundational change: Software and workflows are streamlined to enable digital capture of diverse data across all areas of R&D.
Transformational change: Multimodal data from various sources are harmonized and collectively analyzed to uncover key insights.
Aspirational change: AI drives in silico predictions and discoveries that are reality-checked and refined in the lab as part of an iterative R&D cycle.
Dotmatics LumaTM is here to help usher in these changes.
Powering the Lab-in-a-Loop with Dotmatics Luma
Dotmatics Luma is a multimodal scientific platform that unites science data with data science to deliver an AI-powered Lab-in-a-Loop. As shown in the figure below, the Dotmatics approach to Lab-in-a-Loop centers on the marriage of the wet and dry labs, with Luma at the core. Experiments can be simulated and modeled in silico and then used to inform the next tests in the wet lab—ultimately creating a continuous, intelligent learning cycle.
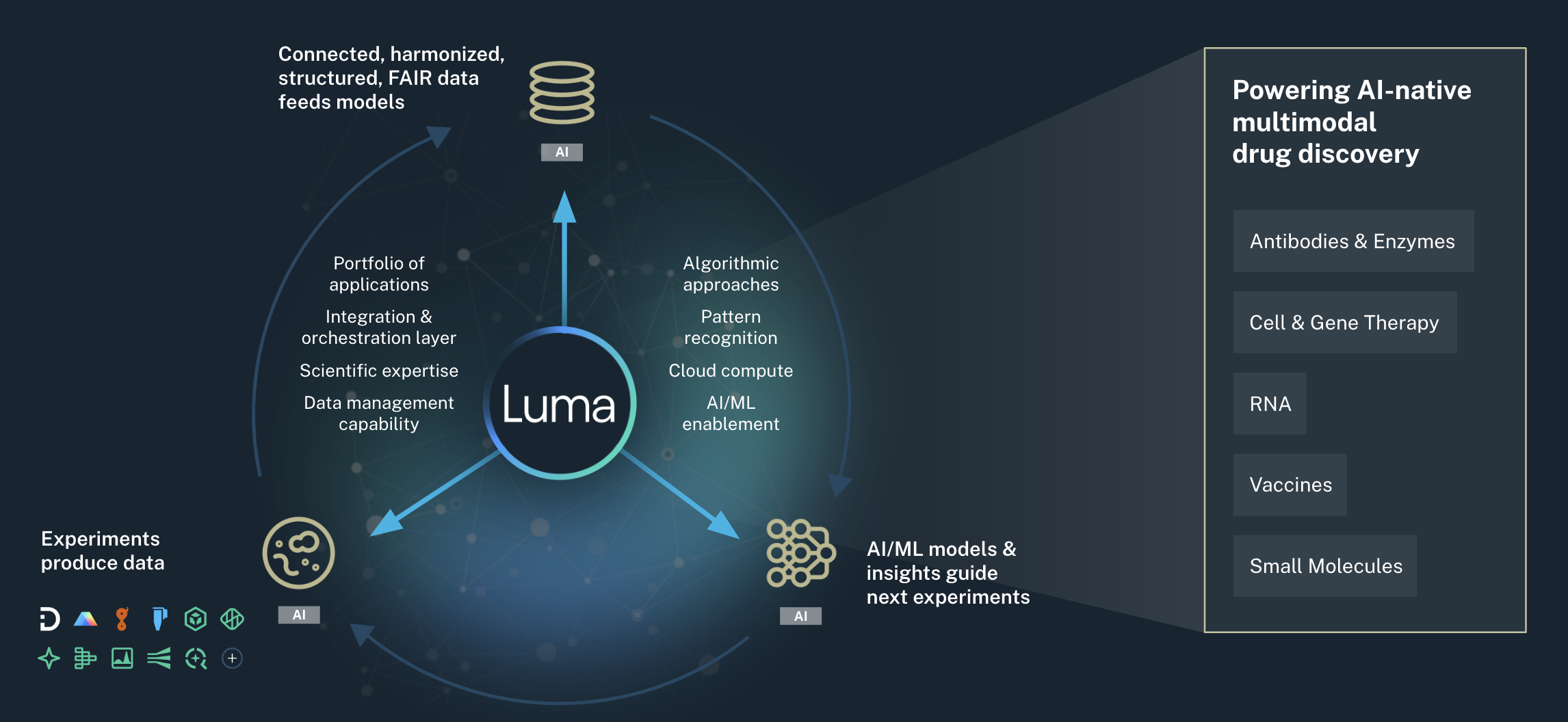
The data foundation made possible with Luma lets:
Scientists and administrators unify and analyze vast volumes of multimodal data for better decision-making.
Researchers pull in data from all of the most popular scientific applications, databases, and lab instruments—a significant development for scientists who typically struggle with critical data trapped in silos.
Teams process R&D data of incredible volume and complexity at a nearly exponential scale, with scientists empowered to take control on their own, without constant IT intervention.
By using Luma to create a Lab-in-a-Loop, teams can achieve the multidirectional data flow needed to train and refine predictive AI models that help inform and optimize lab innovation. This Lab-in-a-Loop approach will radically transform the notoriously convoluted drug discovery process and empower companies to deliver life-changing therapeutics to patients in a faster, more cost efficient, and increasingly personalized manner.
Learn More About Luma
Luma is helping companies of all sizes and specialties achieve the digital transformation needed to make AI-driven R&D a reality.
Request a demo to see how Luma can transform your R&D processes by bridging bench science with in silico innovation.