Part 2: Putting Data First in Considering a Scientific Informatics Platform
In our last article, we considered the many different challenges that must be factored into thinking about what a comprehensive science informatics platform needs to do. These challenges boil down to huge scope and multiple dimensions of organizational complexity. The purpose of this exercise was to frame the opportunity in its proper perspective and to suggest a way forward: solve the data problem first. In other words, work backwards from the end goal.
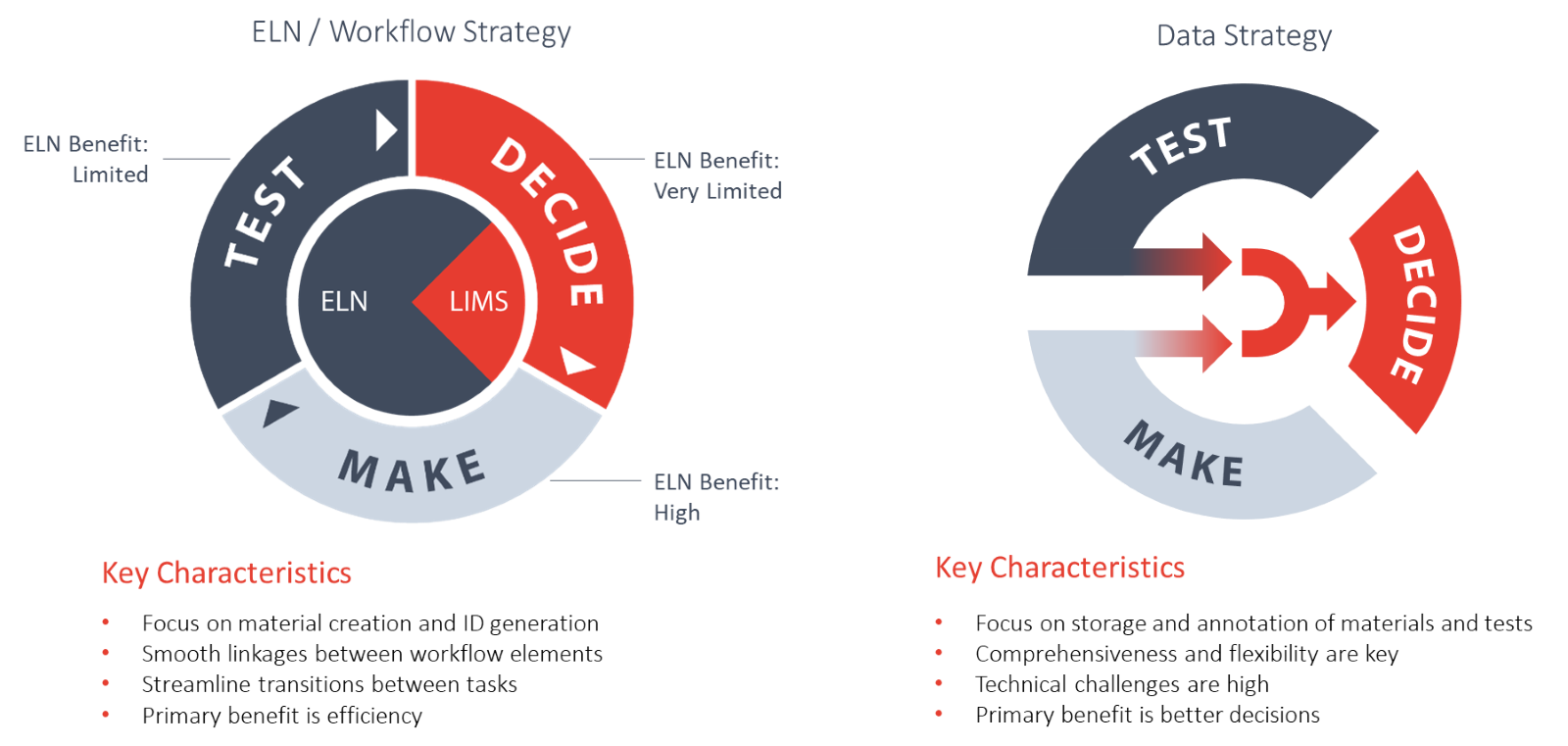
Data-driven Decisions
There is a natural temptation to start with workflow before data flow. After all, the workflow produces the data. There are also obvious benefits to providing software to facilitate workflow. It increases efficiency. It eliminates repetitive and value-free tasks. It makes scientists feel like they are working in a modern way and being productive. However, if one drills into the requirements behind ELN-first, workflow-oriented solutions, there is always the promise of better decision-making. All the data required for good decisions will be captured in the ELN where it can be put to beneficial uses. It is really the seeming potential of enabling better decision-making that is behind most IT investments, and there is the assumption that a great workflow solution will make this much easier. However, given that much work is performed external to sponsor-provided systems and that most sponsor-provided systems are a patch work of different software components anyway, ELN cannot be the answer to improved dataflow leading to data-driven work processes.
Platform Componentry
Componentry is always required to ingest external data. Given that this componentry is required anyway, it is better to invert the problem and focus on building out a data management platform that can ingest and organize all the data your organization produces. Moreover, while capturing data is hard; mobilizing it quickly and effectively to make better decisions is even harder. Multiple workflow solutions exist to solve individual problems. True, they do not all exist in a common platform, but they do exist. The same is not true of effective data management systems, which are expensive and difficult to either procure or buy and usually require a significant investment in coding, which is both expensive and slow.
The underlying challenge to effective data management solutions is the inherent diversity and scale of scientific data. A straightforward way to think about this problem is that decision support systems are exceptionally good at adding rows of data (scale) but not so good at adding columns of data nor in defining the relationships between them. New ways of organizing scientific data are required that make handing diversity much easier and quicker. Self-service capabilities to organize data much closer to the scientists doing the work. Luckily, innovative technologies exist to make handling the diversity deluge much more tractable. While these technologies are evolving rapidly, there are organizations that have heavily invested in understanding and implementing these novel approaches. Start with the data. Find a partner that has invested heavily in using and deploying them. Go from there.
In our next article, we will consider the different dimensions of what makes a scientific data management platform effective and achievable.